I ride my bike to work so I’m often thinking about the best path to take around corners. I know bike racers and car racers (and bob sledders) are often told to head into a corner wide, then cut the apex, and then exit wide again. Basically the gist is that you want to make your actual path have the largest turn radius possible so that you don’t slip out. The question I was thinking about recently was whether there was some compromise since typically the largest radius path (which allows the largest speed without slipping out) is also the longest path (and hence mitigates a little the fact that it’s the faster path). I also realized that in car racing, and to a limited degree bike racing, the speed is not held constant throughout the path, so I wondered how you could find the optimal path and the optimal speed adjustments throughout. That’s what this post is about.
First a quick story about go-karts. I was “racing” in one (against my friends) and I was trying to follow the wide/narrow/wide path through all the corners. But I was losing! I finally realized that the wheels had terrific grip and that I could floor the pedal and hug all the curves and never spin out. My friends knew this and by the time I figured it out it was too late.
So what’s the physics involved here? The key is to figure out why wheels start to slip in the sideways direction. They have a particular amount of grip and that force provides the instantaneous centripetal acceleration for the wheel. If you know what the grip force is, along with the instantaneous radius of curvature, you can find the fastest possible speed at that section of the road:
or
So, if you know the path of the road, you should be able to figure out the maximum possible speed at every location. So how do you do that? Well, first let’s make sure we understand how we’re mathematically describing the path.
What I decided to do was just pick some random points in the plane. Then I interpolate a path that smoothly connects them all. Here’s the Mathematica syntax that does that:
pts = RandomReal[{-1, 1}, {5}]; intx = Interpolation[pts[[All, 1]], Method -> "Spline"]; inty = Interpolation[pts[[All, 2]], Method -> "Spline"];
So now we have two functions, intx and inty, that characterize what the path does. You can plot the path now using:
ParametricPlot[{intx[i], inty[i]}, {i, 1, 5}]
which give this:
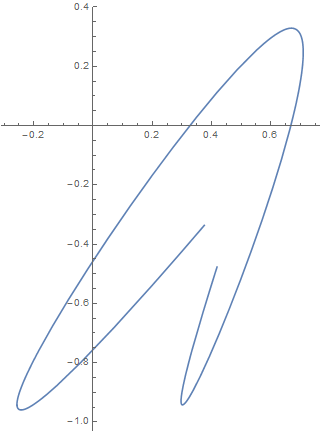
Main path considered in this post
I knew there was likely some cool differential geometry formula for finding the curvature at any point and I found it at this wikipedia page:
which I can calculate now that I have the interpolation functions from above. Cool, so now I can find the radius of curvature at every point:

This shows the instantaneous radius of curvature at every point along the curve.
So now I can use the equation above for the velocity at every point and figure out a trajectory, and more importantly, a time to traverse the path, which I’d love to minimize eventually.
To be clear, I pick an arbitrary grip force and then calculate the radius of curvature and hence the max speed everywhere and I figure out how long it would take to make the journey. I realized that I’d risk the occasional infinite speed for straight portions of the track so I decided to build in a cap on the speed, that I arbitrarily picked.
So how do I figure out the time once I know the speeds. Pretty easily, actually, as for every segment of the path the small time is determined by the distance, divided by the speed:
where again i is the parametrization that I used (it just basically counts the original random points) and the speed (v(i)) is calculated as above.
Ok, cool, so if you give me a path, I’ll tell you the fastest you could traverse it. But that doesn’t yet let me figure out better paths around corners. To do that I need to generate some other paths to test to see if they’re faster. Remember they might not be as tight of turns (and so likely faster at the curves) but they’re then going to be likely longer. The hope is that we can find an optimum.
How do I generate other test paths? Well, for each of the original random points, I perturb the path in a direction perpendicular to the original path (which I’ll start calling the middle of the road). If there’s 5 points, then at each the path will move a little left or right of the center, and I’ll use the spline interpolation again to get a smooth path that connects all those perturbations.
So now it’s a 5 dimensional optimization problem. In other words, what is the best combination of those 5 perturbations that yields a path that allows the car to make the whole journey faster. Luckily Mathematica‘s NMinimize function is totally built for a task like this. Here’s what it found:
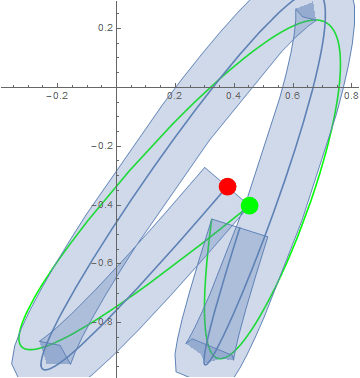
The blue stripe is the road. The blue curve is the middle of the road. The red point travels along the blue curve as fast as it can without slipping. The green curve is the result of the optimization process. The green point moves along the green curve as fast as it can without slipping.
Note how in the last curve the red point has to significantly slow down, allowing the green point to win. Cool, huh?
Here’s another example that I didn’t have the patience to let NMinimize run (I let it run for 30 minutes before I gave up). It took so long because I used 10 original points, and so it was a 10 dimensional optimization problem. Luckily, just by running some random perturbations I found a significantly better path. Note how it accepts a really tight turn towards the end but it still ends up winning:
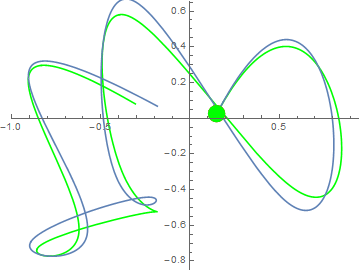
10 dimensional optimization example
As a last note, I should mentions that making the animations took me a while to figure out. I knew the speed at every point (note, not the velocity!) but I needed to know the position (in 2D) at every point in time. I finally figured out how to do that (obviously). Here’s the command:
NDSolve[{D[intx[i[t]], t]^2 + D[inty[i[t]], t]^2 == bestvnew[i[t]]^2,
i[0] == num}, {i}, {t, 0, tmax}]
where tmax was how long the path takes. Basically I’m solving for how fast I should go from point 1 to the last point (i as a function of time). Then I can just plot the dots at the right location at {intx[i[t]], inty[i[t]]}. That worked like a charm.
Alrighty, that’s been my fun for the last few days. Thoughts? Here are some starters for you:
- Wow, this is really cool. What I really like is the . . .
- Wow, this totally blows. What really makes me mad is . . .
- Can I get a copy of the Mathematica document?
- Why do you set the initial condition on i to be at the last point instead of the first? (editors note: that took me a long time to get to work, luckily the paths calculated are time reversable)
- What do you mean they’re time reversable?
- I race for a living and these are way off. Instead what I do is . . .
- I want to race for a living now that you’ve given me the tools to win. Where do I send my royalty checks?
- It seems to me that the cap on the speed gives you discontinuities in your acceleration. Is that allowed?
- I don’t get your NDSolve command at all. What is that differential equation?
Hi, Andy. If the speed varies, the friction force must provide both the centripetal and tangential acceleration components, and it’s the magnitude (not just the centripetal component) that has to stay below the static maximum. Though perhaps the tangential component is always small enough to ignore?
yeah, great point Ian. I would think that the tangential would be small, especially at the apex of the curve, but I’ll give it a try calculating it to see.
Cool exploration and great post so my suggestion is an epsilon of change: since we want to know who wins the race, could you freeze the last frame in the animation for, say, 1 sec before it loops? Then we’d see who wins and by how much.
Peter
Really nice post. Really nice. However, I’m still wondering about the original problem. If you’re headed into a 90 degree turn on your bike, and all options are available (no traffic), what path should you take?
Pingback: Help us crowd-source our drums! | SuperFly Physics